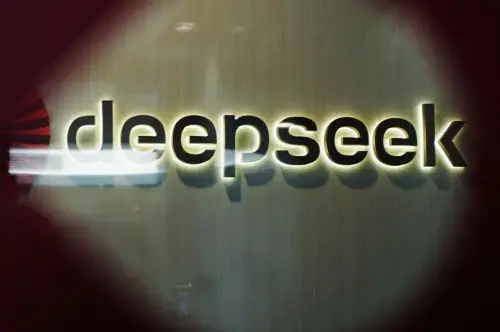
DeepSeek, a Chinese artificial intelligence startup founded in 2023, has quickly made waves in the global industry. With fewer than 200 employees and backed by the quant fund High-Flyer ($8 billion in assets under management), the company released its open-source model, DeepSeek R1, one day before the announcement of OpenAI's $500-billion Stargate project in the United States.
What sets DeepSeek apart is the prospect of radical cost efficiency. The company claims to have trained its model for just $6 million using 2,000 Nvidia H800 graphics processing units (GPUs) versus the $80 million to $100 million cost of GPT-4 and the 16,000 H100 processors required for Meta's LLaMA 3. While the comparisons are far from apples to apples, the possibilities are valuable to understand.
DeepSeek's rapid adoption underscores its potential impact. Within days, it became the top free app in US app stores, spawned more than 700 open-source derivatives (and growing), and was onboarded by the Microsoft, Amazon Web Services and Nvidia AI platforms.
DeepSeek's performance appears to be based on a series of engineering innovations that significantly reduce inference costs while also improving training cost. Its mixture-of-experts architecture activates only 37 billion out of 671 billion parameters for processing each token, reducing computational overhead without sacrificing performance.
The company also has optimised distillation techniques, allowing reasoning capabilities from larger models to be transferred to smaller ones. By using reinforcement learning, DeepSeek enhances performance without requiring extensive supervised fine-tuning. Additionally, its multi-head latent attention (MHLA) mechanism reduces memory usage to 5% to 13% of previous methods.
DeepSeek's hardware and system-level optimisations further enhance performance. The company has developed memory compression and load balancing techniques to maximise efficiency. As well, it has improved the communication between GPUs using the DualPipe algorithm, allowing processors to communicate and compute more effectively during training.
So far, the results aren't surprising; indeed, they track with broader trends in AI efficiency. What is more surprising is that an open-source Chinese startup has managed to close or at least significantly narrow the performance gap with leading proprietary models.
Despite DeepSeek's claims, several uncertainties remain. The true cost of training the model remains unverified, and there is speculation about whether the company relied on a mix of high-end and lower-tier GPUs. Questions have also been raised about intellectual property concerns, particularly regarding the sources and methods used for distillation.
Some critics argue that DeepSeek has not introduced fundamentally new techniques but has simply refined existing ones. Nevertheless, boardrooms and leadership teams are now paying closer attention to how AI efficiency improvements could impact long-term investment plans and strategy.
AI MARKET SCENARIOS
DeepSeek's impact could unfold in several ways. In a bullish scenario, ongoing efficiency improvements would lead to cheaper inference, spurring greater AI adoption. While inference costs drop, high-end training and advanced AI models would likely continue to justify heavy investment, ensuring that spending on cutting-edge AI capabilities remains strong.
A moderate scenario suggests that AI training costs remain stable but that spending on AI inference infrastructure decreases by 30% to 50%. In this case, cloud providers would reduce their capital expenditures from a range between $80 billion and $100 billion annually to a range between $65 billion and $85 billion per service provider, which would still represent a 2-to 3-times increase over 2023 levels.
In a bearish scenario, AI training budgets shrink, and spending on inference infrastructure declines significantly. Capital expenditures for cloud providers could drop to a range between $40 billion and $60 billion, which would still be 1.5 to 2 times higher than 2023 levels.
Amid the speculation, some observations may help put events into context:
Significant leap, not surprising: Inference costs have been steadily declining, and DeepSeek's innovations accelerate this trend rather than disrupt it entirely.
Don't overreact: AI adoption will continue expanding robustly, though the pace and shape of investment may shift.
Inference is only one slice: The largest players are still racing to build next-generation models that unlock frontier applications and a bigger total addressable market.
Impact by segment: An intensified arms race in the model layer, with open source versus proprietary forming up as a key battleground, sees short-term volatility and medium-term strength in data centre hardware and app players benefitting.
Energy demand: Near-term demand through 2030 is unlikely to change materially given power supply constraints; longer-term implications remain uncertain.
Overall, demand for AI capabilities remains strong. Data centres, hardware providers, and AI application developers will continue evolving as efficiency improvements unlock new possibilities.
THE CEO PLAYBOOK
For CEOs, the DeepSeek episode is less about one company and more about what it signals for the future of AI. The lesson is clear: The pace of AI innovation is rapid and iterative, and breakthroughs can come from unexpected places. Executives can take three key steps:
Avoid overreaction, but prepare for cost disruption. DeepSeek's model may not be an existential threat to AI incumbents, but it highlights the rapid decline in AI costs. Businesses should plan for a world where AI inference is significantly cheaper, enabling broader adoption and new competitive dynamics.
Monitor market signals closely. Keep an eye on capital expenditure trends, GPU demand and AI adoption rates. If infrastructure spending slows, it could indicate that efficiency gains are reshaping AI economics.
Think beyond productivity -- AI as a business model catalyst. The real winners will be those that use it to redefine their core offerings, not just cut costs.
Peter Hanbury is a Partner in the San Francisco office and Jue Wang is a Partner in the Silicon Valley office of Bain & Company.