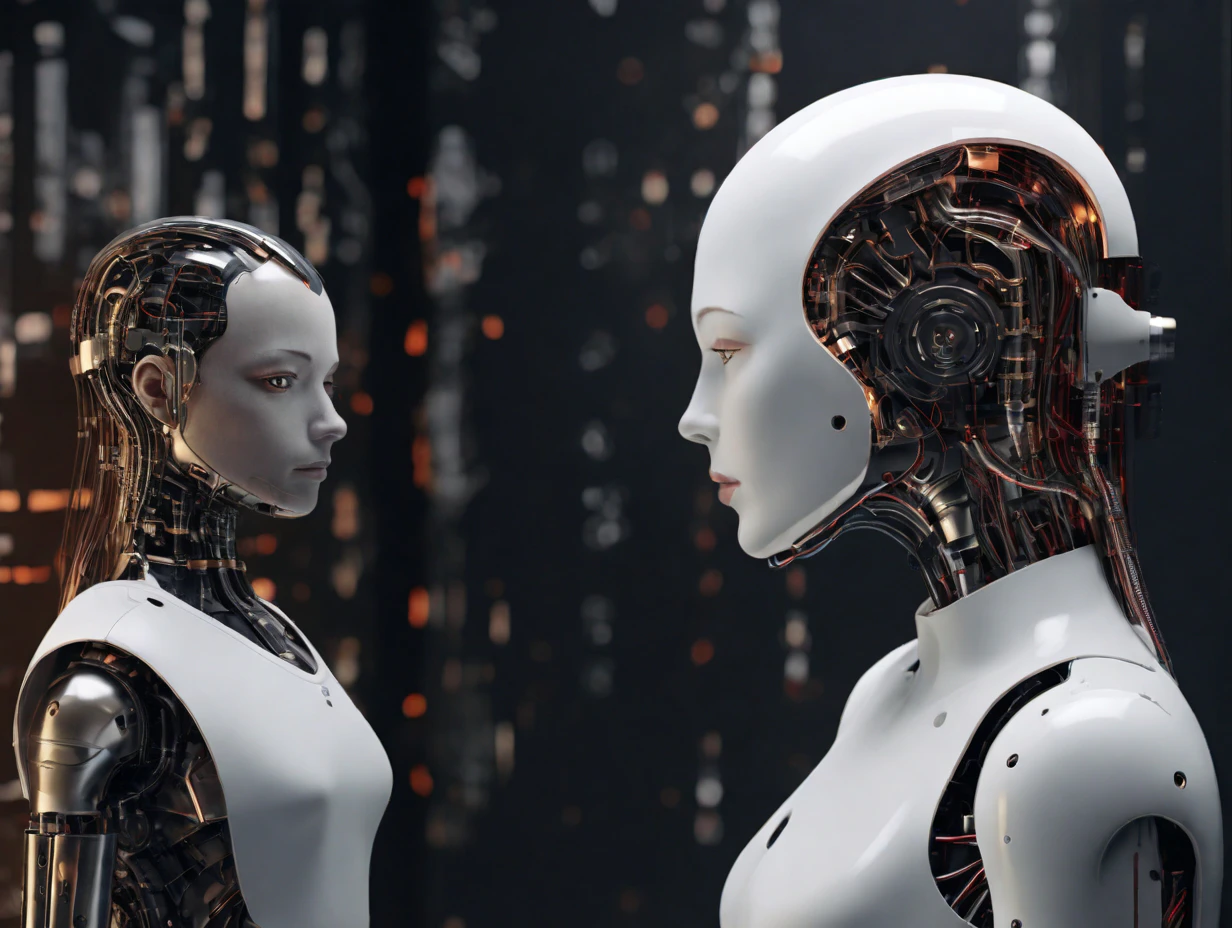
As organizations increasingly embrace artificial intelligence (AI) to enhance their quality assurance (QA) processes, they face many challenges. From data dependency to ethical considerations, navigating the complexities of implementing AI in QA requires careful consideration and strategic planning.
Navigating complexity: Understanding the black box
Implementing AI for QA introduces a significant challenge: complexity. AI models, often regarded as “black boxes,” operate with millions of parameters, making it challenging to interpret their inner workings. This opacity can hinder troubleshooting efforts when issues arise. However, solutions such as utilizing models with transparency features, like attention maps or decision trees, offer insights into AI’s decision-making process, aiding in understanding and troubleshooting.
The effectiveness of an AI model hinges on the quality of its training data. Organizations must meticulously evaluate and curate datasets, ensuring they are representative and free from biases. Furthermore, privacy concerns necessitate anonymizing sensitive data to adhere to regulatory requirements. Organizations can bolster the reliability and integrity of their AI-driven QA processes by prioritizing data quality and privacy compliance.
An essential aspect of implementing AI in QA is striking the right balance between automation and human insight. While AI streamlines processes and detects patterns, human judgment offers contextual understanding and nuanced decision-making. Achieving this balance involves benchmarking AI outputs against human expertise, ensuring that AI augments rather than replaces human intuition in the QA process.
Embracing AI in QA requires addressing skill gaps and providing comprehensive training to employees. By conducting skill assessments and developing tailored training programs, organizations can effectively equip their workforce with the necessary knowledge and expertise to leverage AI. Various training formats, including online courses and mentorship programs, facilitate continuous learning and skill development, enabling employees to harness the full potential of AI technologies.
Cost implications: Evaluating investments in AI tools
Adopting AI in QA entails significant financial investments, encompassing acquiring AI tools and the infrastructure to support them. Organizations must evaluate the cost implications and ROI of integrating AI into their QA processes, from expensive data training systems to AI platform licenses. Balancing cost considerations with the potential benefits of AI-driven QA is crucial for strategic decision-making and resource allocation.
Explainability and transparency are paramount when implementing AI in QA. Utilizing AI models that offer clear decision-making processes, such as decision trees or rule-based systems, enhances transparency and facilitates understanding. Additionally, leveraging tools like SHapely Additive explanations (SHAP) and Local Interpretable Model-Agnostic Explanations (LIME) provides insights into AI’s decision-making rationale, fostering trust and confidence in AI-driven QA processes.
Ethical and legal considerations loom large in the realm of AI-driven QA. Biases within AI models can lead to legal ramifications, potentially violating anti-discrimination laws. Moreover, intellectual property rights and data privacy necessitate meticulous adherence to regulatory frameworks like GDPR and CCPA. By proactively addressing ethical and legal considerations, organizations can mitigate risks and ensure compliance in their AI-driven QA initiatives.
Testing AI systems: Adopting rigorous testing techniques
Testing AI systems poses unique challenges, necessitating innovative techniques like adversarial AI and mutation testing. Adversarial AI exposes vulnerabilities by creating modified outputs designed to deceive AI models, while mutation testing evaluates models’ responses to unexpected inputs. Organizations can identify and address weaknesses in AI-driven QA systems by adopting rigorous testing methodologies, enhancing reliability and robustness.